Defining Your Data Governance Strategy for 2024

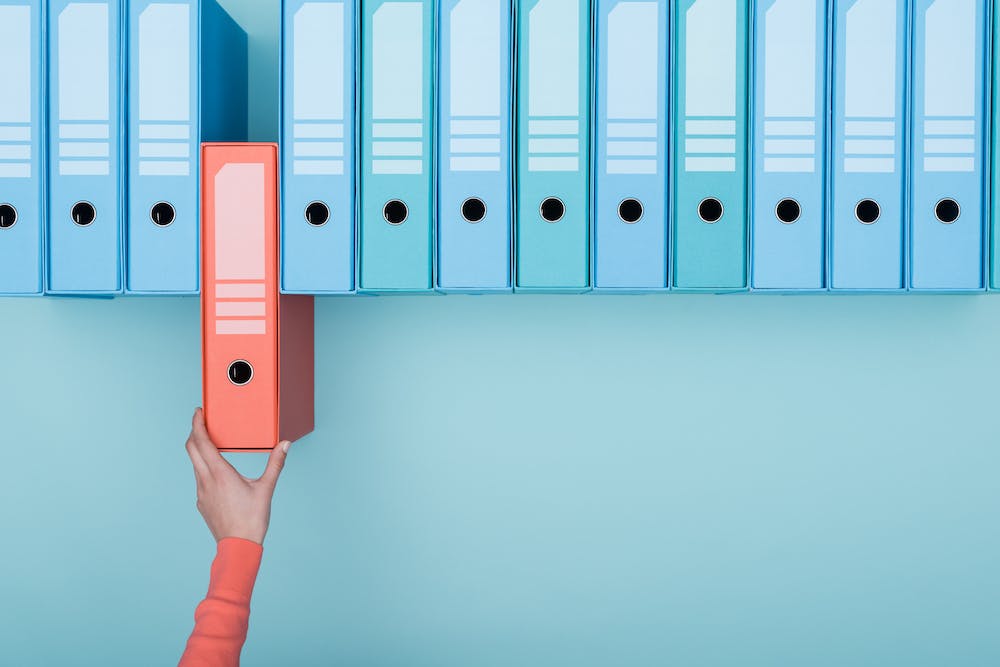
The effectiveness of your data governance program is determined by how much care and attention you put into the design of its strategy. A well-designed governance strategy sets a sturdy foundation for your framework to click into.
Rush, or worse, skip this step, and your entire data governance structure could collapse.
This post outlines a six-step process to mapping out a well-thought-out data governance strategy that will give your data governance program the highest chances of success.
A data governance strategy is an organization’s plan for using, protecting and managing data throughout the entire data lifecycle.
This complete scope of influence is addressed through six primary data governance objectives:
Minimal Sensitive Data Access - Implement access control mechanisms, like Least-Privilege, ensuring users can only access the minimal level of sensitive data required to perform their daily duties.
Data Access Observability - Ensure all data access is always logged by recording who accessed what, where and when.
Regulatory Compliance Tracking - Ensure perpetual audit readiness by keeping data records up-to-date and data processes aligned with regulatory standards.
Data Security - Ensure ongoing protection against unauthorized access with encryption and user verification protocols.
Data Retention - Implement policies clearly stipulating retention periods for all data types, and across all sensitivity levels.
Sensitive Data Accountability - Maintain a catalogue of all your data flows, categorized by type and sensitivity level.
But a data governance strategy isn’t just sustained by a framework. It’s driven by a collaborative effort including people, technology, and processes, all bound together by centralized policies and management guidelines tailored to the business’s unique data context.
Figure 1
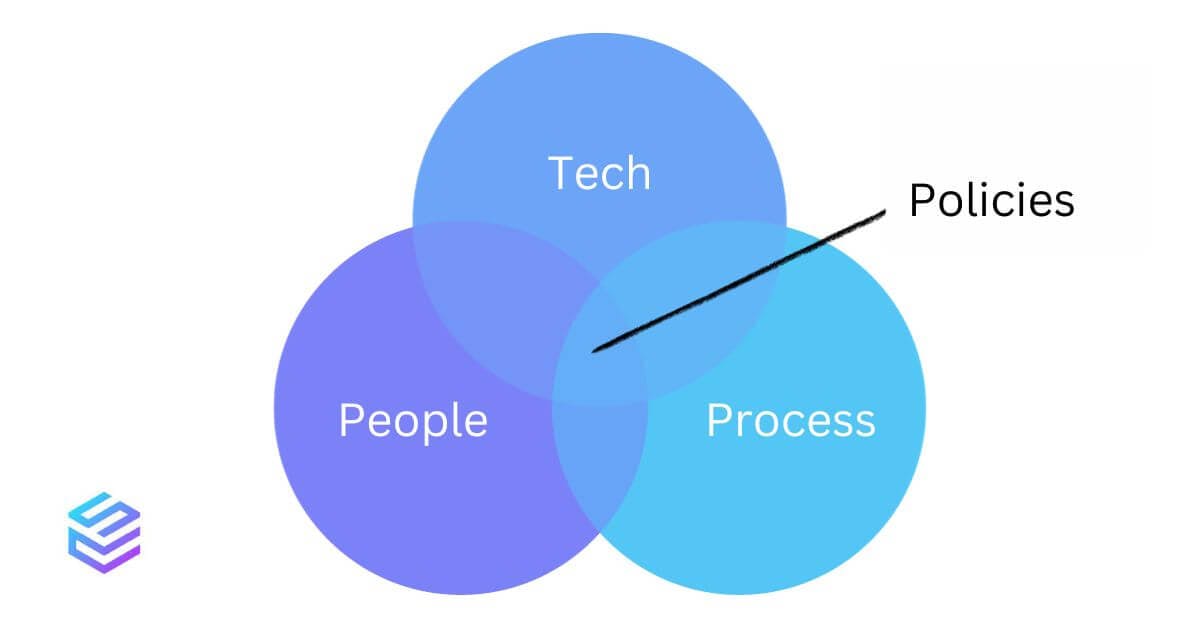
Why is a Data Governance Strategy Important?
The consequences of poor data governance are significant. Limited data awareness increases the likelihood of sensitive data slipping through the cracks in your security controls, resulting in a disastrous data leak - like the one suffered by multi-national security service provider, Securitas.
In October 2021, the cybersecurity team at SafetyDetectives discovered nearly 3TB of sensitive data belonging to Securitas which was exposed to the public through a leaky Amazon S3 bucket. The misconfigured bucket was used to store the Personal Identifiable Information (PII) of security staff in Columbia and Peru, along with photos of fueling lines, planes, and metadata revealing the GPS locations of each photo.
This leaked information could have provided sufficient intelligence to arm a terrorist threat if it had fallen into the wrong hands.
Securitas closed the bucket five days after SafetyDetectives informed them of the leak. In the dynamic and fast-paced world of cybercrime, five days is a very long time to keep a vulnerability exposed to the public. Collectively, we don’t know how long the leak was active or whether threat actors had accessed any data during that time.
Could a data governance strategy have prevented this breach?
Absolutely!
Clearly defined access control policies and procedures would have restricted access to this treasure trove of sensitive data to privileged users, and a strong data security policy enforcing encryption-in-use would have kept this data continuously encrypted, and therefore, of no use to cybercriminals while stored in the cloud.
Figure 2
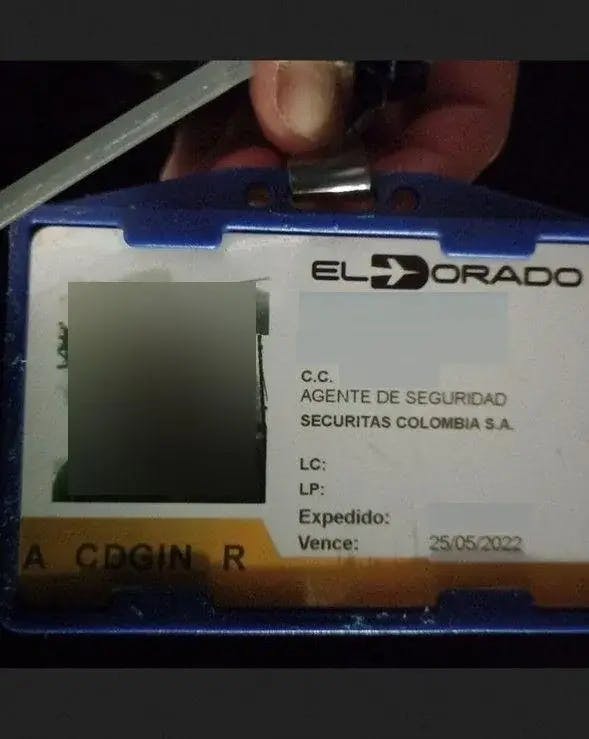
A Securitas employee’s ID exposed in the Securitas data leak - Source: SafetyDetectives.com
6 Step Guide: Defining Your Data Governance Strategy
A mistake many organizations make when approaching data governance is to jump straight into the implementation of a framework. That's like commencing construction work without waiting for the concrete to set.
Before even thinking about the concept of frameworks, you need to identify your unique data management objectives and define a governance strategy mapping to these objectives. Only after this point are you ready to move on to framework design and implementation.
A data governance strategy outlines how data will be managed across the organization. A data governance framework is then implemented to execute this strategy.
Figure 3
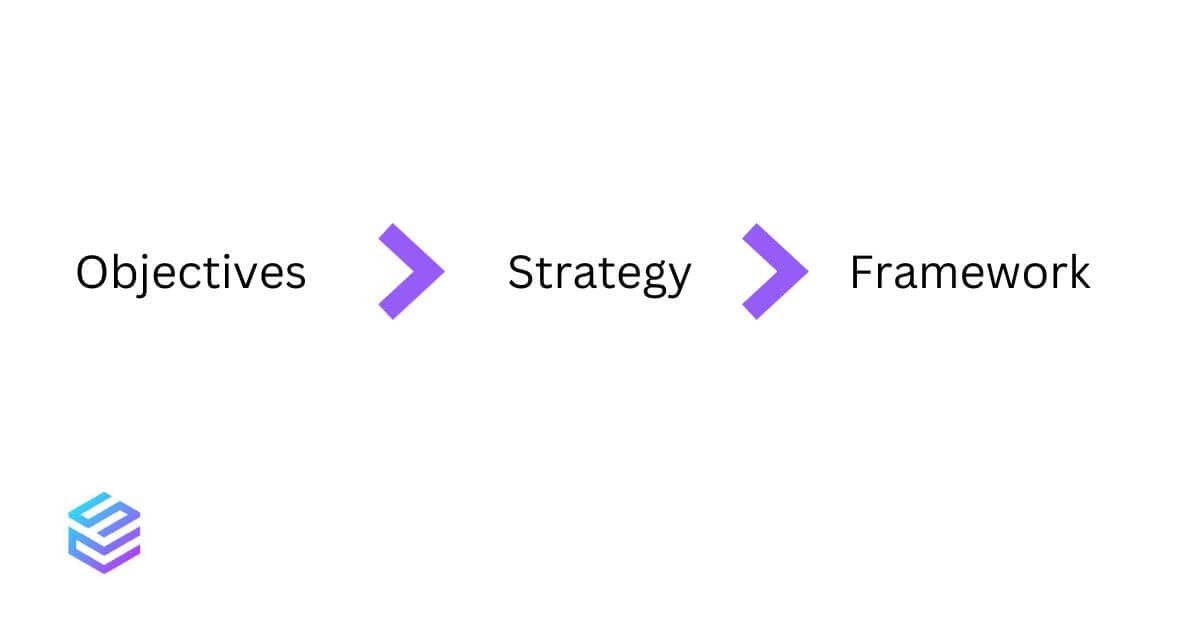
The following steps will encourage you to approach the implementation of a data governance program in an ideal sequence, beginning with the thorough design of a governance strategy.
Step 1: List all Factors Impacting Data Governance
This is where you begin understanding your baseline data management context by outlining all the factors in your organization impacting a prospective governance strategy.
To get the ball rolling, use this example list of internal and external factors below as a template. Use insight-gathering methods such as assessments and surveys to collect data in each category. Then, use this information to map your business’s internal and external data governance factors.
After completing these profiles, you should know how accommodating your current data management context is to a data governance strategy - information that will prepare you for the degree of friction you may experience when implementing a governance framework.
Examples of internal factors impacting data governance.
Organizational Culture - The leadership team influences your workplace culture. So, if they don't prioritize data-driven decisions and organizational-wide data literacy, you will likely experience excessive friction while implementing a governance strategy.
Data Quality - Data quality levels directly impact the quality of data-driven decisions. This factor has greater relevance if your organizational culture considers data an asset for strategic thinking (see point above).
IT Infrastructure - The data storage, integration, and management platform aspects of your IT infrastructure could be designed to either hinder or support data governance efforts.
Data ownership - Data responsibility and ownership roles that are clearly defined make data governance efforts much easier. Ownership ambiguities create visibility gaps, setting a weak foundation for a data governance framework.
Policies and Procedures - Data governance policies reflect how well an organization’s data is being managed and provide insights reflecting an organization’s baseline level of compatibility with a data governance framework.
Communication Effectiveness - The effectiveness of an organization’s communication channels reflects how efficiently data will be managed in a governance strategy.
Data Security and Privacy - An organization’s baseline level of data security indicates its degree of data visibility and, therefore, its ability to align with the cybersecurity expectations of a data governance strategy.
Metrics and KPIs - Internal metrics measuring data governance performance and efficacy support the continual improvement of a data governance strategy.
Examples of External factors impacting data governance.
Regulations - Applicable data-related regulations like the General Data Protection Regulation (GDPR) or the California Consumer Privacy Act (CCPA) significantly influence your data management storage and sharing efforts.
Industry-Standards - Industry-specific best practices mandating data security standards, like the Health Insurance Portability and Accountability Act (HIPAA).
Third-Party Vendors - Because third-party services are often used to outsource data management and storage processes, their data governance practices will impact the efficacy of your own data governance efforts.
Cybersecurity threat environment - The state of your internal and external threat landscape governs the cybersecurity strategies required to protect your organization’s data.
Globalization - As organizations scale to a global scale, the data governance standards of other nations need to be factored into your own governance strategy plans.
Step 2: Define Your Data Governance Objectives
Now that all the factors influencing your data governance efforts are listed, you can begin outlining your primary governance objectives. These can be broad categories considering your unique data management needs, stakeholder reporting requirements, and technological innovation priorities.
To get the most value from this exercise (and increase your chances of meeting each objective), make goals specific, measurable, relevant to your organization, and include clearly defined deadlines.
Use this example template to inspire the design of your data governance objective list.
1. Data Classification
Specific: Create a standardized classification system for all enterprise data.
Measurable: Classify at least 95% of all enterprise data into appropriate categories.
Actionable: Have the IT manager lead the implementation of a data classification strategy across all departments.
Relevant: A data classification process will simplify governance by enhancing data security and ensuring the most relevant data is always available.
Deadline: End of Q2 2024.
2. Account Security
Specific: Reduce data breach risks
Measurable: Achieve zero account compromises in the next 12 months.
Actionable: Secure all employee and contractor accounts with Multi-Factor Authentication (MFA).
Relevant: Reducing account compromise risks will reduce the risk of data breaches. Deadline: MFA rollout completion by the end of Q1 2024.
3. Employee Training
Specific: Reduce data compromise caused by employee errors.
Measurable: Zero employee-facilitated security incidents in the next 12 months.
Actionable: Train staff on identifying and reporting common phishing and social engineering tactics.
Relevant: Many breaches begin with a phishing attack. Addressing this attack vector will give us the highest chance of reducing the risk of regulatory violations and financial losses resulting from data breaches.
Deadline: Staff training completed by the end of Q1 2024
4. Data Accessibility
Specific: Ensure data is only accessible on a need-to-know basis.
Measurable: Reduce unnecessary data access pathways by 90%
Actionable: Implement a Privileged Access Management (PAM) solution.
Relevant: On average, approximately 70% of employees have access to data they shouldn’t
Deadline: PAM solution to be completely deployed by Q3 2024.
5. Data Compliance
Specific: Ensure all customer data processes are GDPR compliant.
Measurable: Achieve 100% GDPR compliance for all customer records.
Actionable: Perform a GDPR compliance risk assessment to identify compliance gaps.
Relevant: Failure to comply with the GDPR will result in significant fines and possible reputational damage.
Deadline: Complete GDPR compliance assessment by the end of Q2 2024.
6. Data Archiving and Retention
Specific: Update data archiving and retention policies.
Measurable: Reduce data storage costs by 20%.
Actionable: Review and discard outdated or irrelevant data.
Relevant: Efficient data storage is cost-effective and ensures timely data retrieval.
Deadline: Policy review and data cleanup by end of Q4 2023.
7. Data Quality
Specific: Improve data quality by reducing instances of missing or incomplete entries.
Measurable: Reduce missing data fields by 90%.
Actionable: Implement mandatory fields in data entry forms across all data collection points (internal and public-facing).
Relevant: High-quality data improves analytics and decision-making.
Deadline: Changes to be implemented by the end of Q1 2024.
8. Stakeholder Communication
Specific: Increase stakeholder awareness of data governance policies and efforts.
Measurable: Complete four data governance meetings with stakeholders annually.
Actionable: Organize quarterly data governance meetings with stakeholders.
Relevant: When stakeholders are informed, they can be a better support of data governance efforts.
Deadline: First meeting to be conducted in Q2 2024.
Step 3: Map Your Data Flows
Data visibility is the cornerstone of a data governance strategy. But, as Julius Caesar painfully discovered, you can’t govern what you can’t see.
Before implementing a governance framework, you must evaluate your baseline level of awareness, or better phrased as the baseline level of data ignorance. Shifting the perspective to focus on the negative will program your mind to notice issues, preventing you from getting distracted by what you’re doing well.
You won’t discover cracks in the foundation of your data governance strategy by focusing on what you do well.
The effort of understanding the dynamics of your data flows inside your IT architecture is known as Data Mapping; it involves tracking metrics such as:
Data access requests by users
Data sources access
Data fields access
Data collection controls
External party data access
Resource access - CPI and Memory utilization
Autoscaling
Sources of data integrations
Given the immense complexity of modern digital ecosystems (especially in enterprise architectures), the process of data flow mapping is very tedious and time-consuming. To expedite your understanding of your internal data flows, consider using data auditing software.
At a high level, a data mapping framework consists of four stages.
Identify how your data is shared - Note all the points of data interaction between parties. This could involve sharing data with departments, people, resources, stakeholders, etc,. During this process, you might discover obvious compliance violations, such as collecting personal data without consent. Be sure to document these discoveries.
Identify who has data access - List all users and entities being granted data access, including contractors, third-party services, employees, cloud servers, etc.
Identify all sensitive Information - Take note of all sensitive data and attribute a criticality rating (for simplicity, use a three-tier rating system - Critical, Medium, and low).
Identify security risks - Identify security gaps in each stage of the data lifecycle (for example, storage in a potentially leaky Amazon S3 bucket, and any security concerns in each step of the data handling process (for example, access to sensitive resources by users without privileged access).
A data flow map should be completed at each of these stages. There are many data flow mapping techniques you can use for this. Here are some examples (each item links to a resource with more information):
Data Monitoring
Your completed data flow maps will reveal the level of visibility in your current digital context, which also reflects the potential efficacy of a data monitoring initiative - the process of continuously reviewing data to ensure its ongoing integrity, availability, and usefulness. Gaps or inconsistencies in your data flow maps should be considered red flags, warning you of the ineffectiveness of a data monitoring solution implemented in this context.
A real-time data-monitoring solution should only be implemented when you’ve achieved a confident understanding of every step of your data’s journey, from its creation to its final destruction. Skipping over or rushing this step will ultimately result in your data monitoring solution spitting out erroneous information, which could significantly impede the success of a business driven by data-backed decisions.
Such potential foundational cracks are only discovered if data governance is approached in its intended sequence by first taking the time to outline a strategy before implementing a framework.
Step 4: Complete a Data Security Audit
Get an understanding of your baseline of data security with a comprehensive internal audit. The insights from a data security audit will reveal your level of performance across the following data security best practices.
1. Data Encryption
Data encryption is the foundation of a data governance framework. Encryption methods should feed into all governance processes, protecting your data flows from compromise, even if it slips through a security control gap.
Figure 4
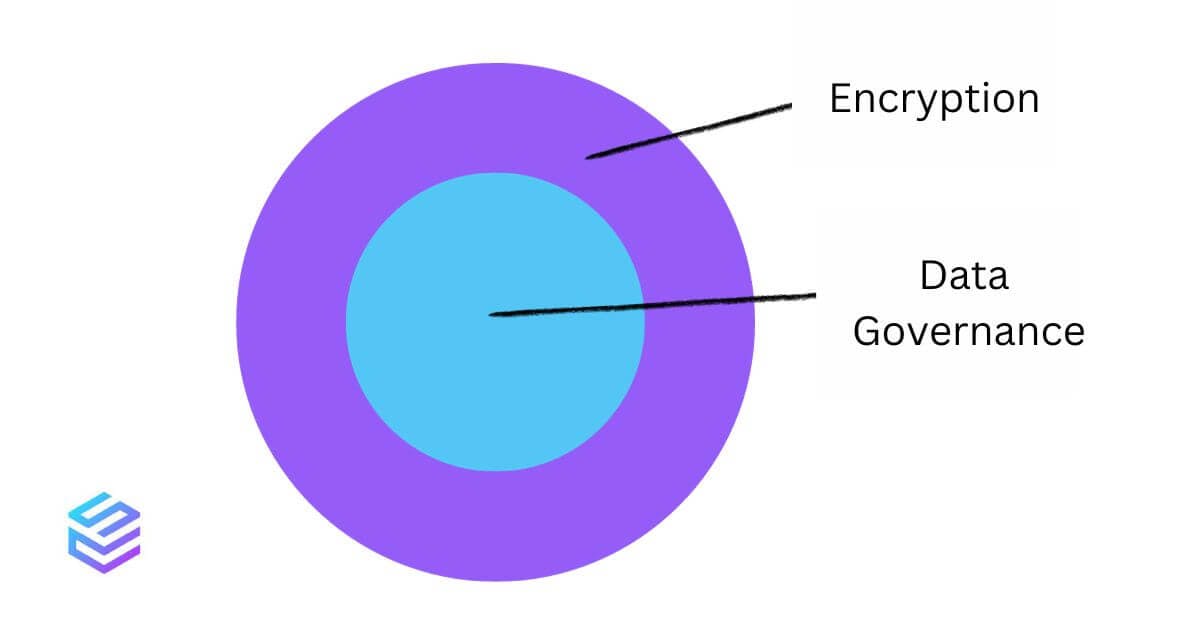
If encrypted with a secure encryption method, exposed data may not be considered a data breach since it’s illegible and useless to cybercriminals.
Encryption at rest - an encryption method explicitly applied to data in storage, isn’t very effective in a governance strategy because, in the dynamic digital ecosystem of just about every enterprise on the planet, data is rarely at rest.
For your governance strategy to be as effective as possible, utilize an encryption-in-use solution - a more modern encryption method applied to data in all phases and across all stages of its lifecycle.
For all encryption efforts discovered in your data security audit, take note of the specific encryption method and all included data resources.
Step 5: Complete an Audit of Your Current Data Management Policies
You likely already have some policies outlining how data should be managed in your organization. These policies should be revised to ensure alignment with the data governance objectives you specified in step two.
Ideally, for the most comprehensive coverage, you should have a policy for each primary stage of your data lifecycles.
Some examples of common data governance policies and their relationship with data classification efforts, are listed below.
Privacy and Protection - Sensitive data should be classified in terms of increasing criticality. This will streamline the application of appropriate protective measures. For example, PII data should be classified as “critical” or “confidential.”
Regulations - Data impacted by regulations should be classified in a unique category. For example, HIPAA regulates the management of patient health records, so PHI data shouldn’t be mixed with non-health-related data.
Data Access - Segregating public, internal, and confidential makes it easier to manage authorized access.
Audit readiness - A proper data classification system streamlines data audits, removing the need for manually processing collected data into ordered categories.
Archiving - Well-classified archived data streamlines access to specific data types when it’s required in the future. Being able to identify and access archived data readily could also support regulatory compliance.
Retention - Different regulations have different retention compliance. Proper classification will help you keep track of the specific regulations associated with each data type and its required retention period.
Destruction - Similar to retention policies, data destruction processes are also governed by regulations. Proper data classification will help.
Step 6: Refine and Adapt Your Data Governance Strategy.
These steps won’t necessarily be completed linearly. You may need to go back and repeat certain processes to confirm and refine alignment with your organization’s governance objectives. For example, while completing your data flow diagrams, you might notice a data processing step not previously accounted for, like a third-party data integration, which would prompt a revision of your set data governance objectives.
Each iteration of this strategy should further tighten alignment with your data governance objectives. Once all discrepancies have been resolved, you can begin strategizing a data governance framework.
Your data governance strategy will only be successful if it remains aligned with your personal data governance objectives.
Given the rapid volatility of the business landscape (especially in recent months), your business objectives will likely change at some point in your journey, and your data governance strategy should be capable of adapting to these changes.
A continuous improvement approach creates a cyclical data governance lifecycle. In this circular lifecycle, data monitoring insights are compared against your current business objectives. When discrepancies are discovered, or your objectives are modified, this triggers cascading changes, starting with your governance strategy and then feeding into your data governance framework. The cycle is ongoing, ensuring persisting alignment with your business objectives, even when they change.
Figure 5
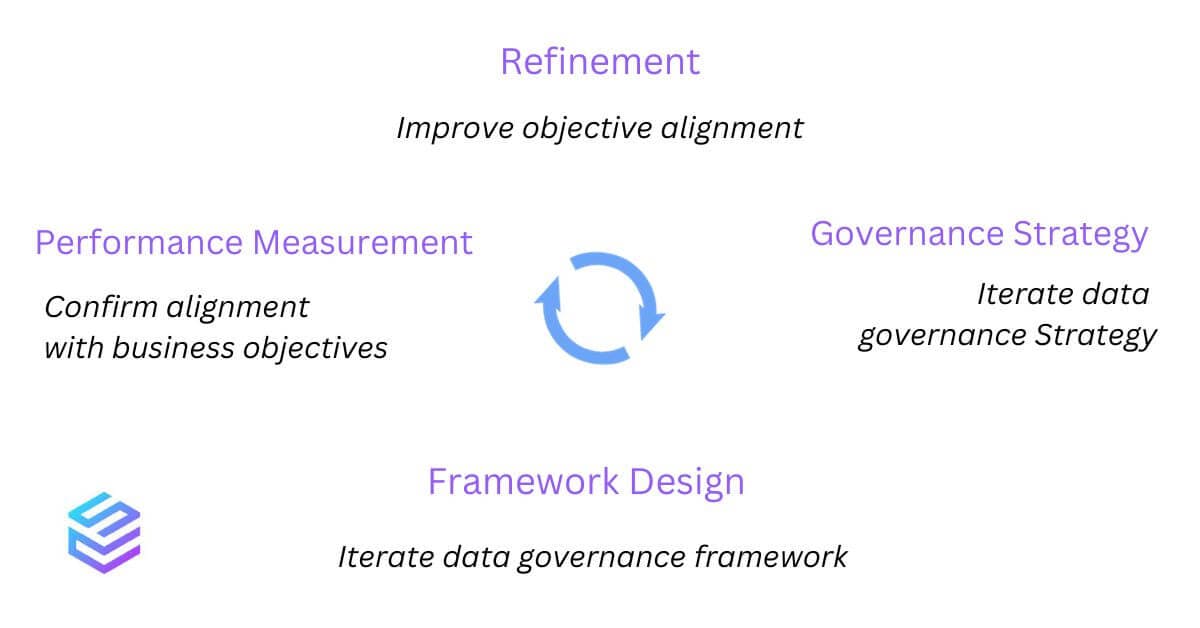
Searchable Encryption by CipherStash
CipherStash keeps your customer data searchable by authorized processes, even when completely encrypted, giving your PII the highest degree of breach protection.